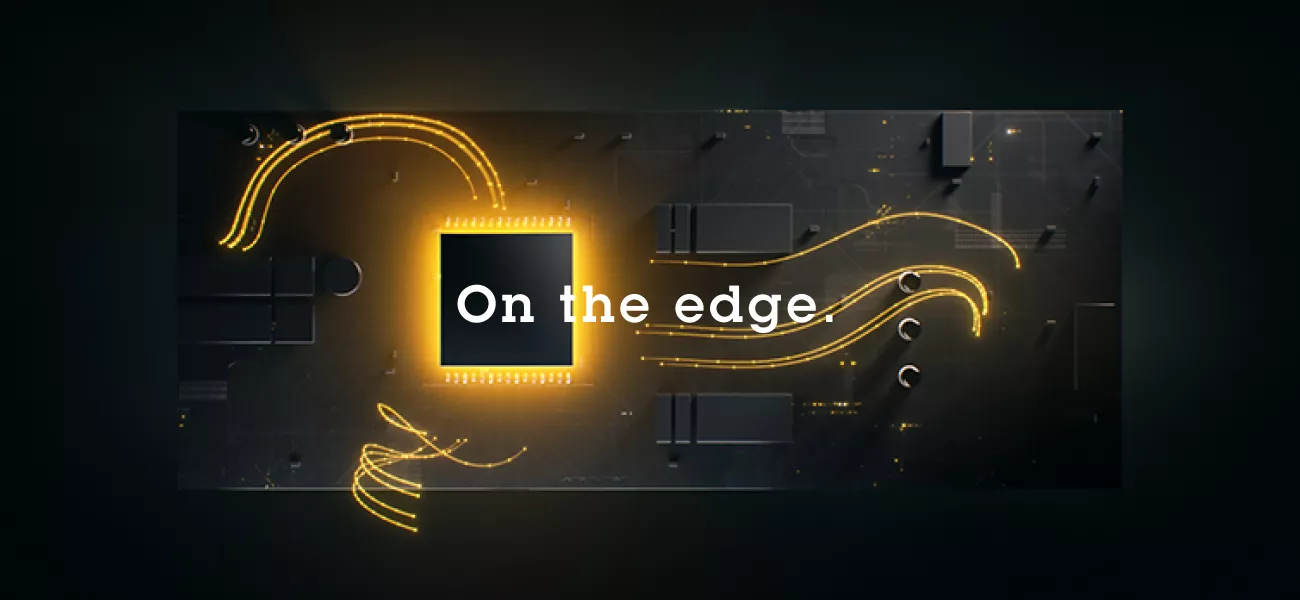
Although it may sound like tech jargon, combining edge computing and AI offers real benefits. Indeed, edge AI is one of the primary areas of where AI is being applied in the security industry, and therefore leading the thinking in how AI is implemented in a responsible way. In this post, we look at the current state of edge AI and the benefits it brings.
Firstly, what do we mean by edge AI?
Before we expect you to accept ‘edge AI’ as a legitimate term, it’s worth an explanation.
In recent years, the number of connected devices on the edge of private and public networks has grown annually, from just under 9bn in 2019 to an estimated 29bn in 2030.
Many of these devices – including surveillance cameras - have also become powerful computing devices. This has resulted in the ability to carry out more processing within edge devices themselves, something which has become established as edge computing.
In line with this increased processing power, advances in machine learning (ML) and deep learning (DL), both subsets of artificial intelligence (AI), have allowed us to bring AI capabilities within the cameras themselves. Hence, ‘edge AI’.
The implications of edge AI in video surveillance
For a video surveillance network, put simply, edge AI means more actions can be carried out on the cameras themselves, largely through more advanced analytics.
AI enables cameras to understand a scene and categorize important details in real-time. This capability to detect, classify, count, and track objects such as humans and vehicles with high accuracy and detail forms the foundation for deriving insights and taking action based on the captured data.
These insights will reduce the burden on the human input required to analyze data and make decisions. Ultimately, it should speed up response times and provide valuable insights that can shape the future of our buildings, cities, transportation systems and more.
Enabling more actionable insights
Edge AI brings significant improvement in capabilities. Previously, motion-based analytics would pick up that ‘something’ has moved and triggered an alert. AI-based edge analytics will identify the movement as a vehicle, classify its type, whether it’s entered a restricted or dangerous area, if there are people nearby who might be in danger, and numerous other elements.
Such information brings far more actionable insight to operators and vastly reduces false positives.
For example, edge AI analytics could identify objects on a motorway (being able to quickly distinguish these from shadows, which has often been a challenge) and automatically alert drivers through signage.
But the additional ability brought through AI to distinguish between a human and a vehicle can help define the level of severity of warning issued to drivers. If cameras saw that there was someone in danger on the road, they could automatically activate signage to slow or even stop traffic and alert emergency services.
Having identified the vehicle (and/or other objects), analytics would also be able to track it, supporting operators in monitoring and investigating an incident.
Enhanced search capabilities
This, alongside the data (and metadata) created by the edge AI analytics, also supports much more advanced searches in video footage. This allows operators to quickly pinpoint specific objects of interest from hours of footage across numerous cameras. Doing so significantly increases the speed and effectiveness of post-incident investigations.
Sharing the load: Edge AI in a hybrid solution architecture
Edge AI brings powerful analytics to the edge of the network, but also plays a valuable role in hybrid solution architectures. Such an architecture makes best use of edge, cloud, and on-premise server environments.
When analytics are centralized on a server, adding more cameras increases data transfer and requires additional servers to handle the analytics. Deploying edge AI analytics means that only the most relevant information is sent across the network, reducing the burden on bandwidth and storage.
Cloud- and server-based analytics can often benefit from preprocessing on the edge, supporting the ‘off-camera’ analytics with more easily digestible metadata. Other analytics might need to be run fully in the cloud or on the server due to their complexity.
Hybrid solutions are taking metadata created by edge AI, feeding this to cloud-based data dashboards, and applying powerful server-based analytics to identify and respond to trends, patterns and anomalies.
Working smartly across a hybrid architecture using the capabilities, capacity, and strength of each part of the system helps to avoid bottlenecks, keeps costs lower, and the performance high.
Edge AI analytics to boost accuracy
In what might seem like something of a ‘meta’ twist, edge AI analytics are now being used to support accuracy as there is no degradation of data.
A key benefit of edge analytics is that the analysis is taking place on the highest-quality video footage, as close as it can be to the source. In a traditional model – when analytics takes place on a server – video is often compressed before being transferred, with the analysis therefore being undertaken on degraded quality video.
However, edge AI depends on high-quality images, but issues like dirty or blocked lenses, or misaligned cameras can cause problems.
As the name suggests, a new category of edge AI analytics – image health analytics – will continually monitor the quality of the image being captured by surveillance cameras and alert operators when it falls below the threshold required for other edge AI analytics to be most effectively applied to the scene.
Edge AI: powering use cases beyond security
It’s clear that edge AI is bringing value to traditional safety and security use cases, allowing first responders and security personnel to react more quickly and appropriately. Additionally, the detailed data and metadata from edge AI analytics are improving operational efficiency across various sectors.
- City planners are using data to power ‘digital twins’ of urban environments, to test improvements in design, traffic management, and noise control to improve the quality of life for citizens.
- Businesses are using analytics to better manage the energy use within buildings, leading to positive environmental impact.
- In healthcare, education, retail, and beyond, edge AI in surveillance cameras is improving service delivering and outcomes for patients, students, and customers.
And even then, we’re only just scratching the surface of the potential of edge AI.