
The past couple of years have seen the topic of artificial intelligence (AI) become part of the conversation in business and homes around the world. While we’ve been working with AI for many years, with deep learning (DL) technologies significantly enhancing video analytics, few technologies have become as high profile as swiftly as AI has, largely due to the advent of generative AI.
Though many might feel that the term has been overused, it’s useful to recap the use of AI in security today but also the genuine potential for AI in the sector, and some of the areas of issues we need to be aware of and manage.
The use of AI in security and beyond today
AI’s integration into security systems has transformed the industry’s approach to threat detection and response. Deep learning (DL) technologies, a subset of AI, have significantly increased the accuracy of analytics solutions, leading to more reliable and efficient security systems.
Examples of applications that now have reliable performance due to the new technology are:
- Detect and track object movement with greater precision.
- Reliably monitor and alert line crossings in sensitive areas.
- Count objects and individuals to manage occupancy and flow.
- Identify loitering behaviors that may indicate potential threats.
The generation of metadata by AI-powered systems has become a game-changer for forensic searches in Video Management Systems (VMS´s). This metadata serves as a foundation for collecting detailed statistics and insights from scene activities, facilitating a more proactive security posture. With the increased capabilities of edge devices this metadata can be generated by the cameras directly reducing the total cost of a system substantially.
AI’s ability to detect anomalies by analyzing patterns and deviations from the norm has introduced a new dimension to security monitoring. This capability allows security professionals to preemptively address potential threats before they escalate.
These capabilities have opened a broad spectrum of additional use cases beyond the traditional applications in safety and security. The fundamental ability to more accurately monitor the flow of persons, material, and products allow for applications that will directly improve operational efficiency. This example from BMW is a perfect illustration, where it is using the AI capabilities of video surveillance cameras to undertake quality inspections throughout the automotive manufacturing process.
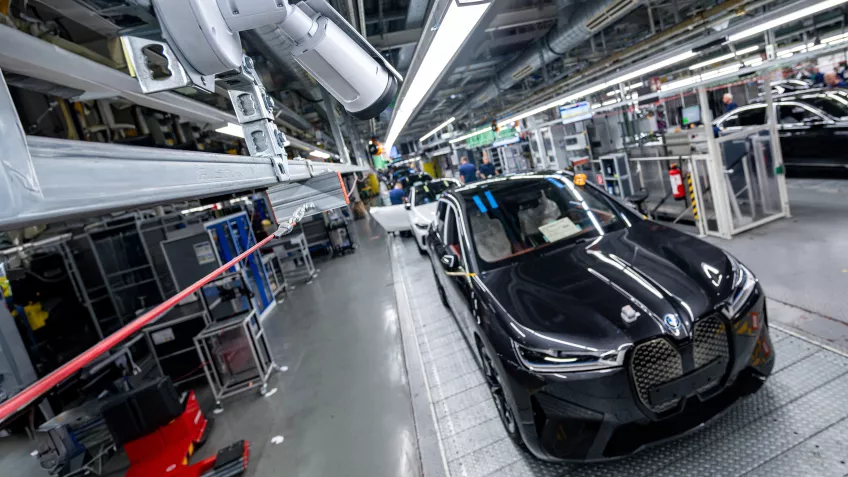
The introduction of generative AI in the security sector
Generative AI, particularly through the use of Large Language Models (LLMs), represents a significant advancement in AI-technology. These technologies enable creation of text and images from natural language prompts. More importantly the huge newer models with their large training data are capable of interacting with users through normal language interfaces, but they are also having capabilities of working with abstract concepts and dealing with complex scenarios.
Generative AI's initial entry into the security sector will be seen by its use in functionalities like:
- Support chatbots that provide real-time assistance to users using natural language.
- Configuration wizards that simplify the setup of complex security systems.
- Text-based searches that enhance the efficiency of data retrieval.
- Advanced design tools that aid in the creation of robust security solutions.
Newer models, so called multi modal models, are able to take text as well as sound, images and video as input and generate results in the form of text and images. This promises to create new possibilities in the security sector where the models are capable of analyzing what is happening in a scene on a new level. The use could include:
- Highlighting significant events that require immediate attention by an operator.
- Analyzing trends and finding common patterns.
- Assisting operators in investigations by providing relevant information and suggestions for action.
- Summarizing video and images for reports
Work to be done to improve accuracy and data quality
Despite the dramatic advancements, generative AI still faces limitations in understanding the nuances of complex scenes and human behavior. The models are still prone to what is referred to as hallucinations, where the model comes up with statements and suggestions that are not accurate.
Another limitation is that the models lack reasoning and logical deduction. These limitations make the use of the models in security critical installations very difficult. We simply can’t rely on that events are not missed or that wrong conclusions are drawn.
Another aspect of using the models in the security context is that we need to manage the risk of biased behavior that the models may show unless the model training data is thoroughly managed.
This underscores the importance of maintaining human involvement in the decision-making process, ensuring that ethical considerations are upheld, and the quality of decisions is not compromised. For this reason, the first use cases where we’ll see LLMs deployed in the security sector will be in providing assistance in forensic search and making suggestions to operators. We will still need to keep the human in the loop. With the rapid pace of innovation we need to find a balance between adoption of new capabilities and mitigating risks of the new technologies.
The potential for AI cameras and edge AI
Any new technology brings challenges to overcome, however. And while we’re still at relatively early stages, it’s clear that AI in the security sector brings significant opportunities to enhance traditional security and safety use cases, while also unlocking huge potential to improve business performance across all industries.
AI cameras delivering edge AI provide the foundation for many of these opportunities, enhancing the accuracy of analytics, enabling scalability of systems and forming the basis for reliable, scalable, and bandwidth-efficient cloud solutions.
The additional metadata created by edge AI analytics, describing in detail the visual data captured by the image sensor, adds further layers of potential analysis and actionable insight. Over time, the aggregation and analysis of metadata will inform decisions that will transform every aspect of an organization’s operations.
The combination of processing within AI cameras, advanced metadata created at the edge, and additional processing in server or cloud, commonly referred to as hybrid solutions, creates a scalable and cost-efficient model for more advanced DL-based analytics solutions.
This creates new opportunities to deliver value beyond traditional security and safety applications. Combining cameras with other technologies like IoT sensors and cloud computing, allows for analyzing visual data in new ways across different areas like cities, transportation, retail, and industrial sectors.
City authorities are combining and analyzing visual and environmental data to enhance the lives of citizens through improvements in air quality, reduction in noise pollution, and better planning of services and infrastructure. Businesses are using data from video surveillance and audio sensors for predictive maintenance of machinery and equipment, creating efficiencies and improving service delivery. Retailers are using store visitor information to improve customer service and store layout. The possibilities are endless.
Innovating, deploying, and using AI responsibly
While AI in all its forms presents significant opportunities, any new technology also carries the potential to introduce new threats and risks. Every technology vendor using AI in its products must recognize its responsibility to develop and deploy AI and other technologies in a responsible manner to mitigate these risks.
Regulation will clearly play its part. The EU has recently adopted the first ever legal framework around AI, The AI Act, and there are other legislations being discussed in the US and many other parts of the world on how to best reduce the potential risks of AI while encouraging innovation. But it’s not enough to follow. Every innovative technology company needs to be driving the responsible and ethical application of AI within its own and its customers’ businesses.
AI and cybersecurity
Cybersecurity and the protection of both data and people’s privacy has long been a focus for the security sector, and AI brings this requirement further into focus. In no small part, this is because cybercriminals themselves will be employing AI in the search for vulnerabilities and new attack vectors. These criminal organizations, well-funded and highly professional as they are, have another advantage in the ‘AI arms race’: they can innovate without consideration for regulations or ethics.
Prioritizing data security and privacy will continue to be paramount. All security technology vendors, but particularly those using AI, must take a human rights-based approach to data governance, ensuring that the collection, processing, and use of data align with human rights principles, fostering a fair, just, and safe digital environment. They also demand the implementation of robust security measures to protect against unauthorized access or misuse and promote data equity by striving for fair and unbiased data representation and access.
There’s also a concern that AI use within surveillance cameras and network devices will create new use cases that raise additional risks and concerns around cybersecurity. Therefore, it is crucial that cybersecurity remains a top priority throughout both the development and implementation phases of new AI solutions.
Unlocking AI’s potential, responsibly and ethically
The opportunities that AI offers to the security sector are exciting. AI can augment human intelligence and the responsible development of AI can benefit people and society. This aligns well with Axis vision, to innovate for a smarter, safer world.
AI’s potential for augmenting our own skills and capabilities will enable us to spend more valuable time on tasks that require our human expertise, making people more valuable than ever.
We must all commit to using AI technology in an ethical and socially responsible manner. This means that AI initiatives, whether related to products and services or ways of working, should be guided by principles of fairness, transparency, accountability, and respect for privacy and human dignity.